Open Sourcing Pinot: Scaling the Wall of Real-Time Analytics
June 10, 2015
Last fall we introduced Pinot, LinkedIn’s real-time analytics infrastructure, that we built to allow us to slice and dice across billions of rows in real-time across a wide variety of products. Today we are happy to announce that we have open sourced Pinot. We’ve had a lot of interest in Pinot and are excited to see how it is adopted by the open source community.
We’ve been using it at LinkedIn for more than two years, and in that time, it has established itself as the de facto online analytics platform to provide valuable insights to our members and customers. At LinkedIn, we have a large deployment of Pinot storing 100’s of billions of records and ingesting over a billion records every day. Pinot serves as the backend for more than 25 analytics products for our customers and members. This includes products such as Who Viewed My Profile, Who Viewed My Posts and the analytics we offer on job postings and ads to help our customers be as effective as possible and get a better return on their investment.
In addition, more than 30 internal products are powered by Pinot. This includes XLNT, our A/B testing platform, which is crucial to our business – we run more than 400 experiments in parallel daily on it.
We chose the name Pinot for two reasons, one obvious and the other a little less so. First, everyone on our team loves Pinot noir. Second, the Pinot noir grape is the toughest of all red varietals to grow and process into wine, yet can produce some of the most complex wine available. This is much like data, which can be so tough to gather and analyze, but so incredibly useful once it's put to work in the right way.
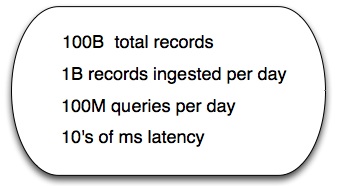
What LinkedIn needed
The need to build rich and interactive analytic products presented some unique challenges. Our critical needs included:
Scalability: Ingest billions of events per day in real time and serve 1000's of queries per second
Low Latency: Site facing applications need response times in the order of milliseconds
Data Freshness: Data must be available for querying in near real time, which means there can only be a lag of few seconds
Fault tolerance/high availability: Resilient to software and hardware failures and continue to meet SLA's
Most companies start with using some of the standard database technologies to meet their initial analytics needs. However scaling is a non trivial challenge as the data size increases. At the time, we could not find any off the shelf solutions that met our requirements like predictable low latency, data freshness in seconds, fault tolerance and scalability.
Design decisions
In order to optimize for low latency on analytic workloads we chose columnar storage for efficient storage and retrieval. Instead of architecting Pinot to accept direct user writes with inbuilt replication, backup and restoration, we designed it as a derived data store. That architectural simplification comes at the cost of a few seconds of data freshness. We ingest data from Hadoop and Kafka. Kafka provides us the ability to ingest data in real time and provide faster insights while Hadoop acts as the source of truth for fact data which allows us to re-bootstrap in case of schema or software changes.
For ease of use we decided to provide a SQL like interface. We support most SQL features including a SQL-like query language and a rich feature set such as filtering, aggregation, group by, order by, distinct. Currently we do not support joins in order to ensure predictable latency. We leveraged Apache Helix as the control plane for cluster-wide coordination.
These design decisions enabled us to build a system that is highly scalable, available and guarantees predictable latency.
Future road map
Another commonly used technique is to pre-materialize data cubes. While this provides low latency and high throughput, it comes with additional storage footprint. We are working on a new indexing technology that is a hybrid of B+tree and Columnar storage. Use cases that need high throughout and low latency will be able to leverage this without incurring high storage overhead.
Getting started
The source code for Pinot is available on Github under Apache 2.0 License. Documentation that covers getting started, design and how to use Pinot is published on the project wiki. We would love to get your feedback on the project.
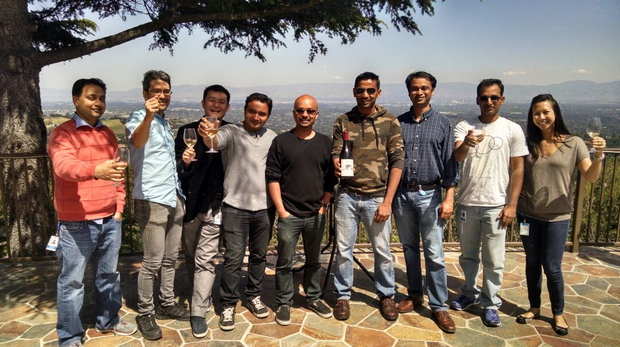
Acknowledgements
This project would not have been possible without the contributions from the Pinot team: Praveen Neppalli, Dhaval Patel, Xiang Fu, Jean-François Im and Mayank Shrivastava with technical guidance from Sanjay Dubey and Shirshanka Das. None of this would run in production without our fearless SREs: Joe Gillotti and Jacob Davida. We would like to thank the LinkedIn engineering, product and business analytics teams who helped in the development of Pinot. We would also like to thank our management Kapil Surlaker, Greg Arnold, Alex Vauthey and Kevin Scott for their support in development and open sourcing of Pinot.